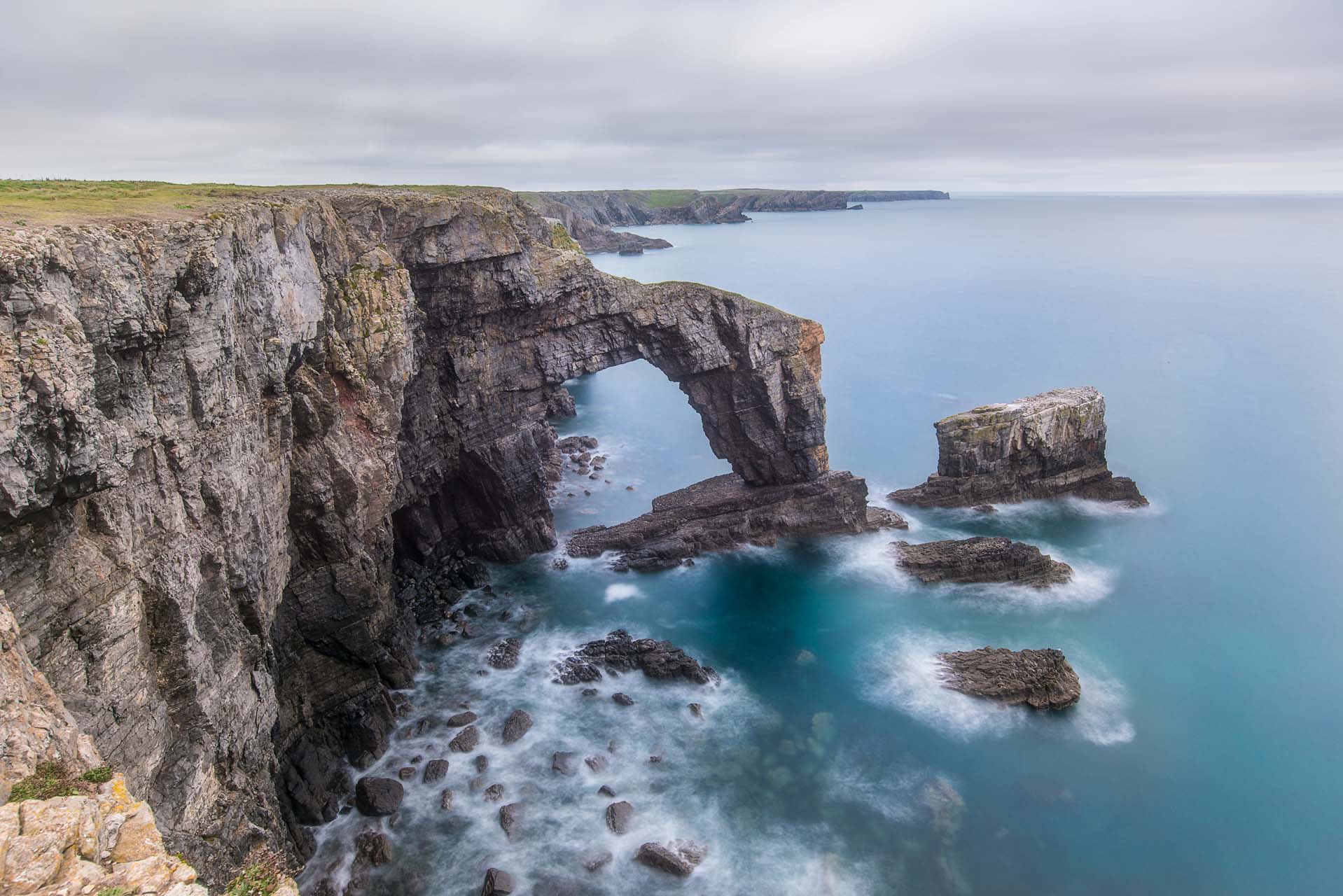
H2020 CEASELESS
Supporting the development of coastal measurements in Copernicus from the Sentinel satellites
Why is it important?
The requirements from end-users and the wide range of applications underpin how crucial the development of oceanographic products is.
The H2020 CEASELESS project is contributing to a better understanding and prediction of coastal oceanography based on developments in satellite and in-situ data as well as high resolution models and novel assimilation approaches.
Project highlights:
Application of new Sentinel data to extract a spatial structure for coastal processes
Application of met-ocean predictions for users as a proof of concept for the new prediction capabilities, highlighting application limits and providing feedback for further developing their coastal dimension
New higher resolution and prediction capabilities for met-ocean variables, to allow for coupling and assimilation at unprecedented scales
In more detail..
The new Sentinel data, together with existing satellite measurements, are supporting a much needed spatial structure to complement the temporal variability captured by in-situ timeseries.
The CEASELESS project has applied the new Sentinel data to selected pilot sites to allow for efficient testing of unstructured grids to better capture coastline irregularities and sea bed geometry and forms.
The pilot sites will allow for more efficient analysis approaches globally, and more specifically in the North Sea and Mediterranean Sea. This serves as proof of concept for the role that coastal processes play in the meteo-oceanographic predictions and the importance of combining in-situ data with satellite measurements for restricted coastal domains.
In CEASELESS, DHI GRAS is acting as the optical remote sensing expert providing Sentinel-2 based satellite-derived bathymetry , water quality data and information on coastal dynamics. Tied up in a cloud-based processing environment a truly scalable approach has been designed capable of delivery of large-scale coverage globally.
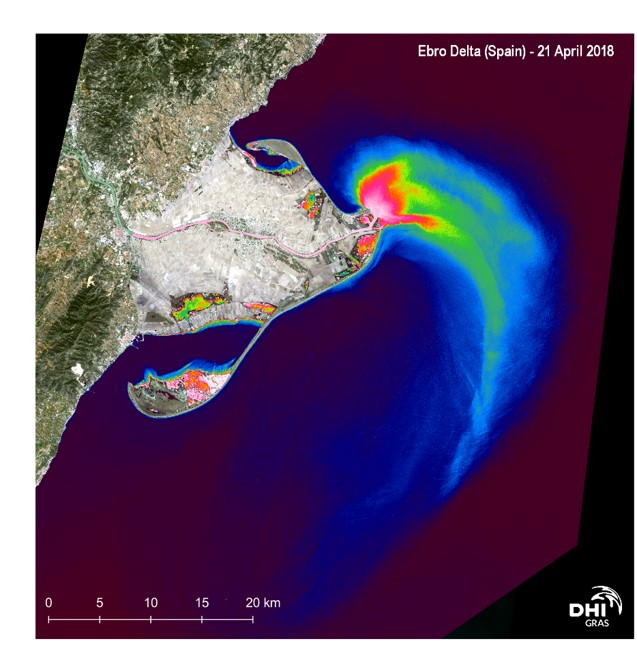
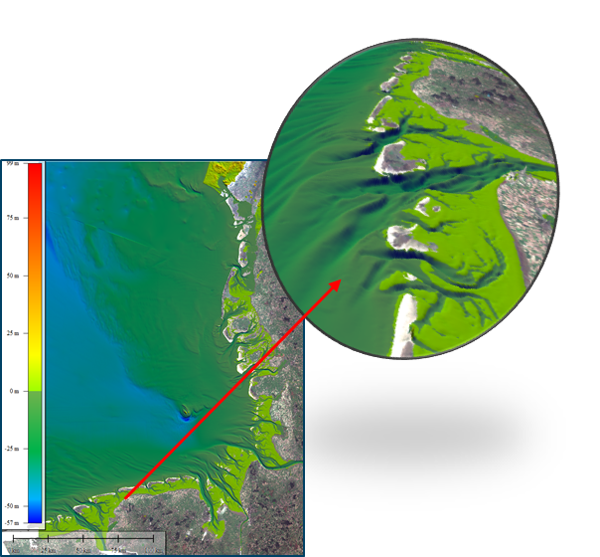
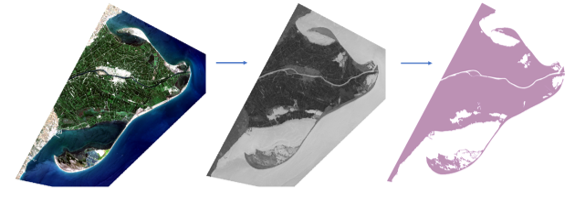
European Commission:
CEASELESS is funded by the European Union’s H2020 Programme for Research, Technological Development and Demonstration under Grant Agreement No: H2020-EO-2016-730030- CEASELESS.
H2020 is the biggest EU Research and Innovation programme and a financial instrument aimed at securing Europe’s global competitiveness by driving economic growth and focusing on research as an investment for the future of Europe.
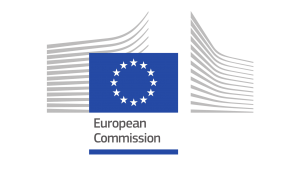
EOatDHI part of the DHI GROUP
gras@dhigroup.com
+45 4516 9100
Agern Alle 5,
2970 Hørsholm,
Denmark
CVR: 36466871